Data-Driven Search and Analysis of Research Software
M.Sc. Florian Sihler
While it is great to see people focusing their life to software engineering and other related fields in computer science, it is important to keep in mind that many of those who have to code or work with code specialize in other domains.
My current research focuses on helping these people with a non-programmer background, especially with the help of static and dynamic analysis tools. For this, I work on a hybrid dataflow analysis algorithm for the R programming language, which is commonly used for statistical analysis. My work received the YoungRSE award at the deRSE24 and the award for the best master's degree in the field of computer science at Ulm University.
If you are interested, feel free to get in touch with me or check out the flowR repository on GitHub.
Furthermore, I assist in teaching:
Useful Links
View the poster here (PDF). FlowR is actively developed on GitHub at flowr-analysis/flowr.
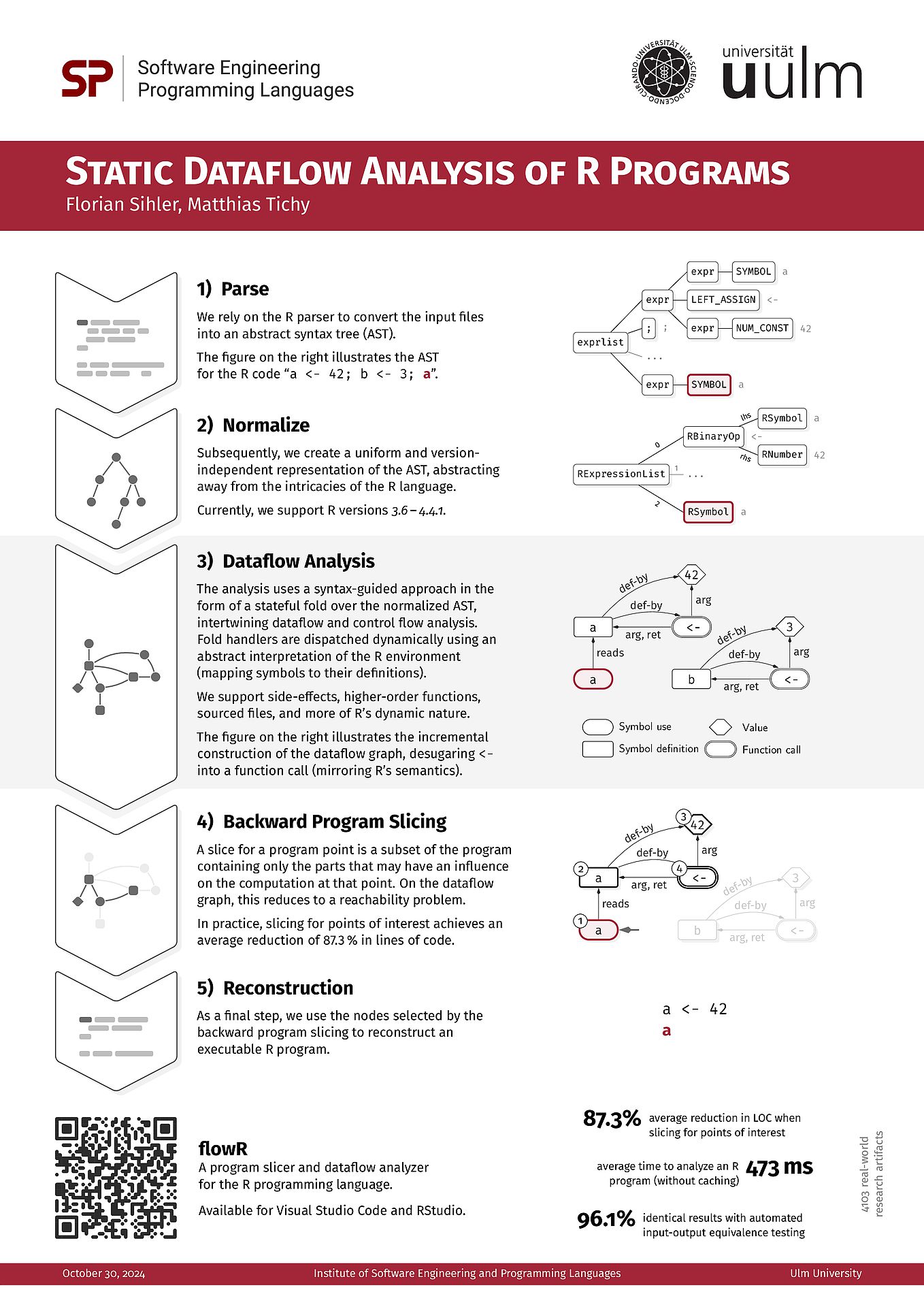
View the poster here (PDF, LaDeWi '24). You can try the game online at: https://exia.informatik.uni-ulm.de/waddle/.
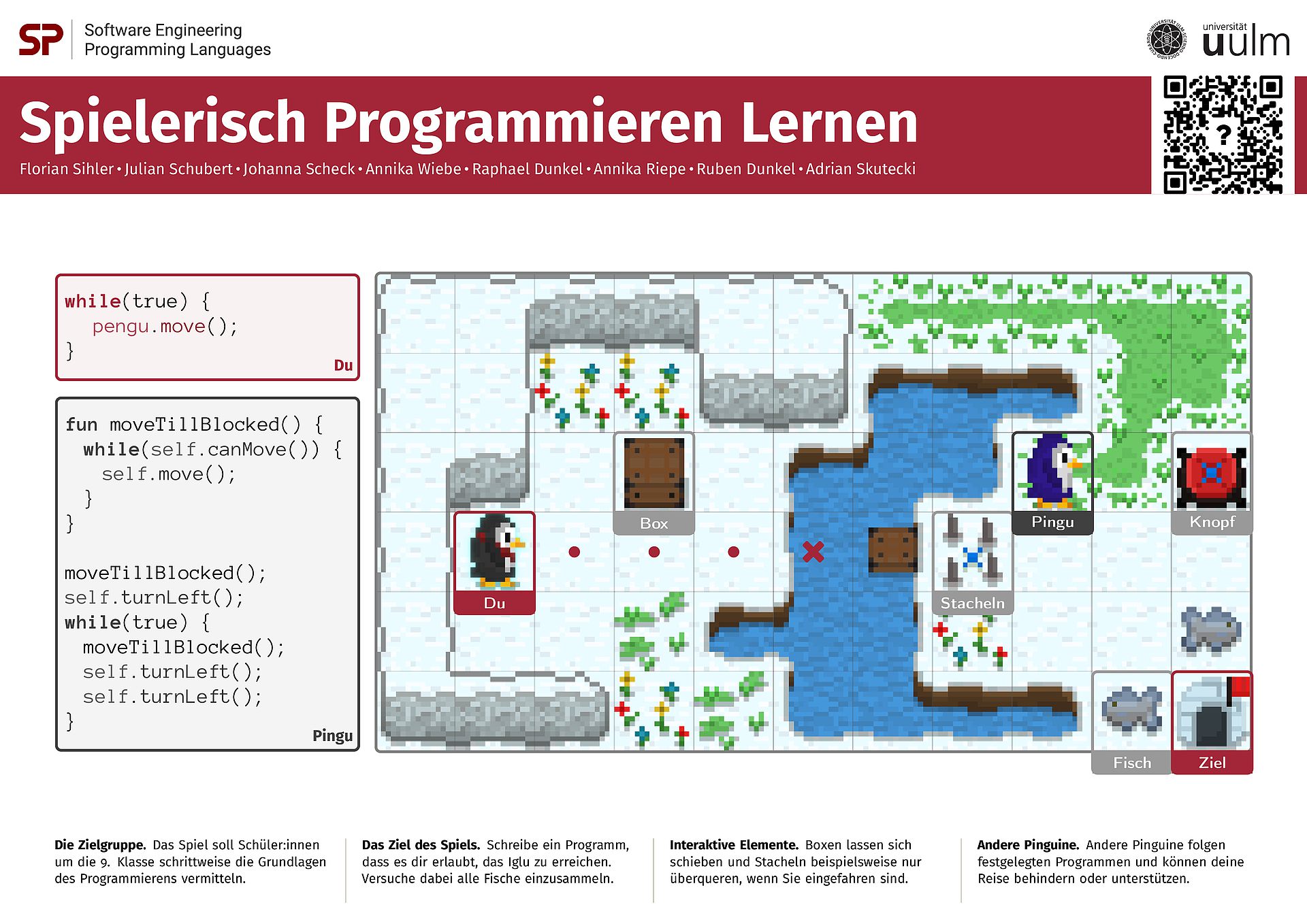
Topics for Theses and Projects
Dynamic and Static Program Analysis
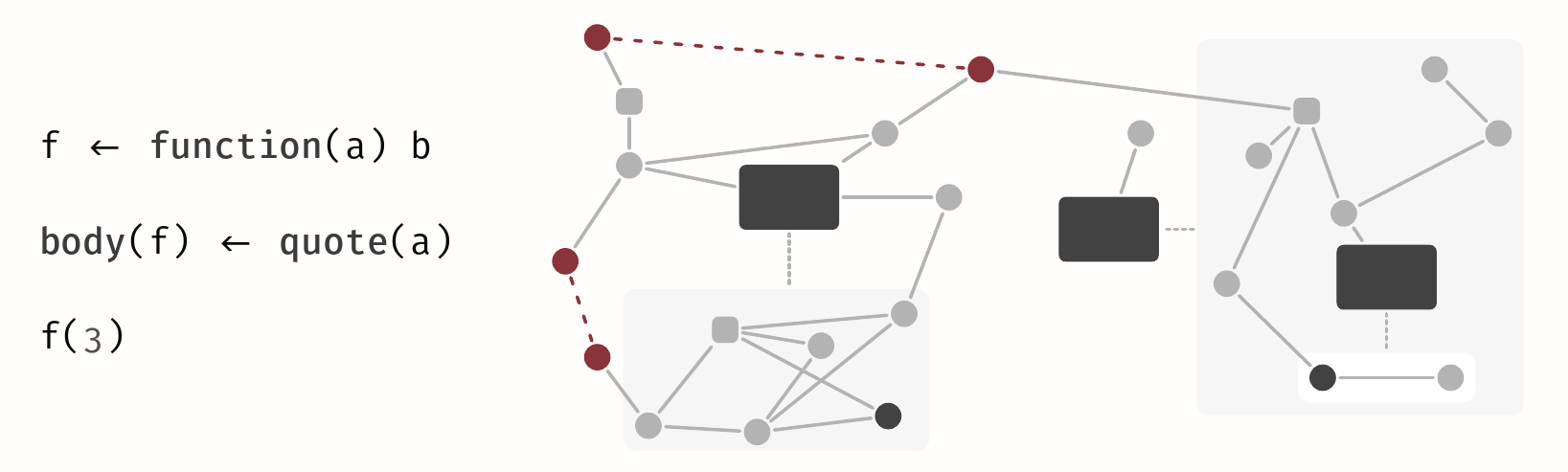
Context
Most static analyzers rely on static dataflow analysis to detect problems like possible null pointer exceptions in code [5].
However, analyzers are usually unable to handle reflective or self-modifying code (e.g., Java Agents, Java Reflection, R's meta-functions [6]). While this is fine for languages in which such constructs are rare or discouraged, they are 1) used quite often in the R programming language, 2) are in-part essential to track program semantics, 3) pose an interesting problem to solve.
Problem
As a basis, we use the static program analysis framework flowR which is designed for the R programming language [3]. flowR is currently unable to deal with reflective and code-modifying constructs like eval, body, quote, and parse in its static dataflow graph.
While handling such constructs statically may be infeasible in the general case, we first want to focus on a set of common cases that appear frequently.
Tasks
- Develop a concept to represent code-modifications and lazy evaluation (within flowR's dataflow graph). For example, to represent a function that has the default values of its arguments or the contents of its body modified.
- Create a proof of concept implementation for this concept in flowR.
Related Work and Further Reading
- K. Cooper and L Torczon. Engineering a Compiler. (ISBN: 978-0-12-818926-9)
- U. Khedker, A. Sanyal, and B. Sathe. Data Flow Analysis: Theory and Practice. (ISBN: 978-0-8493-3251-7)
- F. Sihler. Constructing a Static Program Slicer for R Programs.
- A. Ko and B. Myers. Finding causes of program output with the Java Whyline.
- SonarQube, Sonar.
- Anckaert, B., Madou, M., De Bosschere, K. A Model for Self-Modifying Code.
If you want to, you can have a first look at flowR for yourself: https://github.com/flowr-analysis/flowr.
Contact and More
If you are interested and/or have any questions, feel free to contact me any time.
We can discuss the topic further and try to adapt it to your personal preferences.
Florian Sihler (Institute Homepage)
![[RESERVED] B/M: Automatically Infer Code-Constraints (Sihler, Tichy)](/fileadmin/website_uni_ulm/iui.inst.170/bilder/flowR/code-contracts.png)
Context
Let's suppose you are a data scientist tasked with the analysis of a dataset. As an expert of the domain you have a quick look at the dataset and remember an older script by a colleague which already loads, prepares, and transforms the dataset as you want! Reusing it just leaves you with the task of visualizing the data (as is the common workflow) so you quickly write up and run the script... and luckily realize that even though the script runs and produces figures eerily close to what you would expect, something is not right. The dataset of your colleague never contained a zero so the script contains the implicit assumption of being just able to divide cells.
Within this work we want to make such implicit assumptions explicit in the code, alerting users whenever they no longer hold!
Problem
You have an R script together with the statically inferred dataflow graph that informs you about the control and data dependencies of function calls, variables, and definitions in the program.
The challenges are to
- identify points of interest at which the behavior of the program is defined,
- infer contracts that represent the potential implicit assumptions at the given position (e.g., that the value of a variable has to be non-zero, smaller than the length of another vector, ...), and
- instrument the code to automatically verify these constraints from now on.
Of course, the specific scope of these challenges as well as the focus depends on whether you want to do this as a bachelor's or master's thesis as well as your personal preference.
Tasks
- Enrich flowR [4], a dataflow analysis framework for the R programming language, with the capability to infer predefined constraints
- Create an initial collection of sensible constraints to infer (e.g., non-zero values, ...)
- Infer these constraints and instrument the program to reflect them [5]
One way to infer such constraints would be the definition of abstract domains [1] although classical optimization techniques such as constant folding and constant propagation help as well [2, 3].
Related Work and Further Reading
- P. Cousot. Principles of Abstract Interpretation. (ISBN: 978-0-26-204490-5)
- K. Cooper and L Torczon. Engineering a Compiler. (ISBN: 978-0-12-818926-9)
- U. Khedker, A. Sanyal, and B. Sathe. Data Flow Analysis: Theory and Practice. (ISBN: 978-0-8493-3251-7)
- F. Sihler. Constructing a Static Program Slicer for R Programs.
- B. Meyer, Applying "Design by Contract"
If you want to, you can have a first look at flowR for yourself: https://github.com/flowr-analysis/flowr.
Contact and More
If you are interested and/or have any questions, feel free to contact me any time.
We can discuss the topic further and try to adapt it to your personal preferences.
Florian Sihler (Institute Homepage)
Publications
2024
flowR: A Static Program Slicer for R
ASE '24: Proceedings of the 39th IEEE/ACM International Conference on Automated Software Engineering (Tool Demonstrations)
October 2024
DOI: | 10.1145/3691620.3695359 |
Improving the Comprehension of R Programs by Hybrid Dataflow Analysis
ASE '24: Proceedings of the 39th IEEE/ACM International Conference on Automated Software Engineering (Doctoral Symposium)
October 2024
DOI: | 10.1145/3691620.3695603 |
Exploring the Effectiveness of Abstract Syntax Tree Patterns for Algorithm Recognition
4. International Conference on Code Quality (ICCQ)
June 2024
DOI: | 10.1109/ICCQ60895.2024.10576984 |
ISBN: | 979-8-3503-6646-4 |
On the Anatomy of Real-World R Code for Static Analysis
21st International Conference on Mining Software Repositories (MSR '24)
January 2024
DOI: | 10.1145/3643991.3644911 |
File: |
2023
GenCodeSearchNet: A Benchmark Test Suite for Evaluating Generalization in Programming Language Understanding
GenBench 2023 Workshop
October 2023
DOI: | 10.48550/arXiv.2311.09707 |
Constructing a Static Program Slicer Specifically for R Programs
Masterarbeit
University of Ulm, Germany
August 2023
One-Way Model Transformations in the Context of the Technology-Roadmapping Tool IRIS
Journal of Object Technology
July 2023
DOI: | 10.5381/jot.2023.22.2.a2 |
2022
A domain-specific language for modeling and analyzing solution spaces for technology roadmapping
Journal of Systems & Software (JSS)
February 2022
DOI: | 10.1016/j.jss.2021.111094 |
M.Sc. Florian Sihler
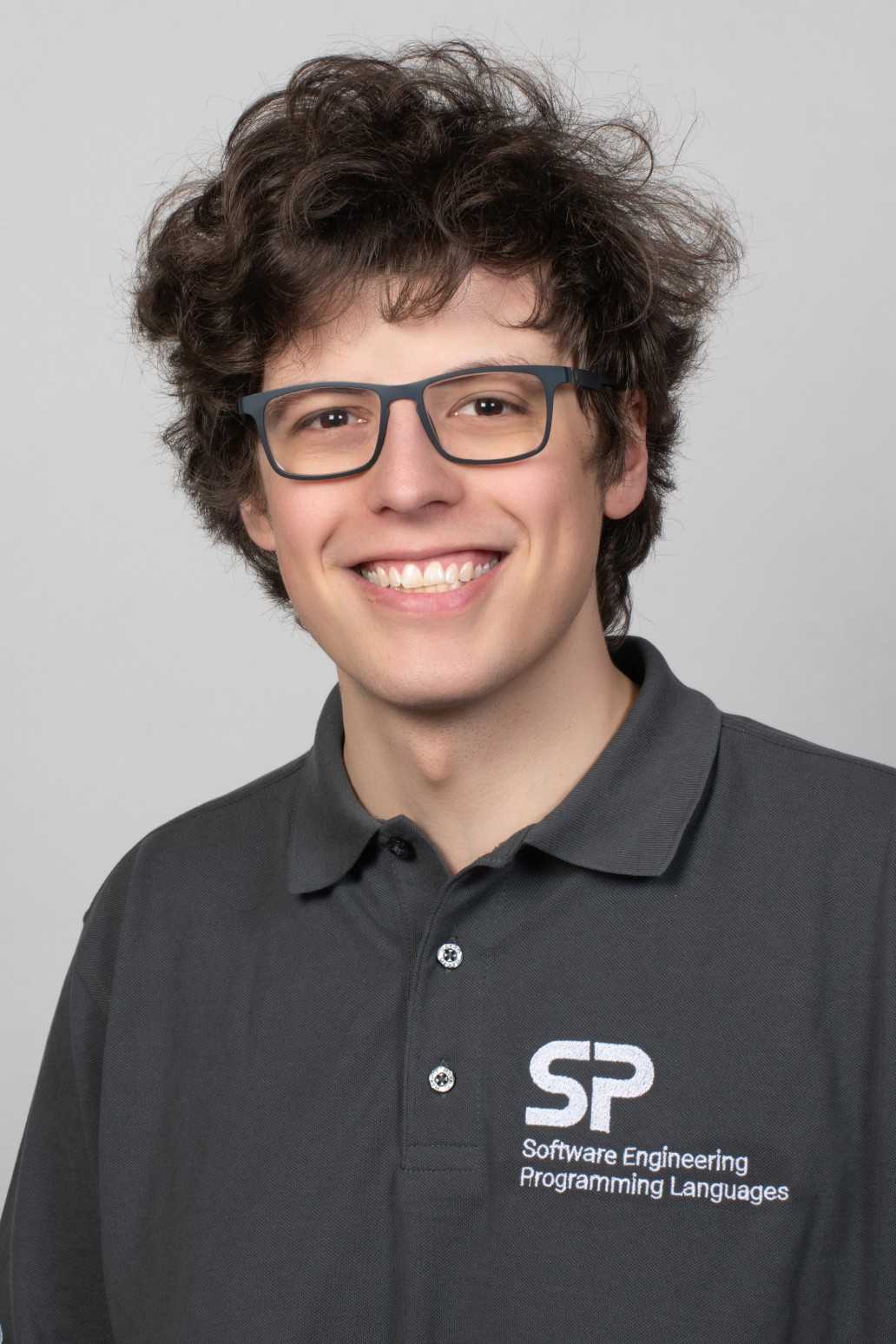
Institute of Software Engineering and Programming Languages
Albert-Einstein-Allee 11