Treatment of patients with tinnitus is designed on the basis of screening data, encompassing answers to question-naires and audiological examination. Since tinnitus affects the quality of life of patients and is associated with comorbidities like depression, the screening step involves the acquisition of extensive amounts of information, which contributes to the design of a personalized treatment. Nonetheless, some patients give up the treatment before completion (“dropout” cases). In this study, we investigate how multi-target classification on the screening data can contribute to characterize patients that will drop out of the study. For our analysis, we observe one more target variable, the “tinnitus loudness”, i.e. the subjectively perceived loudness of the phantom signal, attempting to identify variables that explain this loudness together with the likelihood of interrupting the treatment. We report on our results from the data of 1419 tinnitus patients from the University Hospital of Regensburg.
Studying the Potential of Multi-Target Classification on Patient Screening Data to Predict Dropout Cases
Universität Ulm Universität Ulm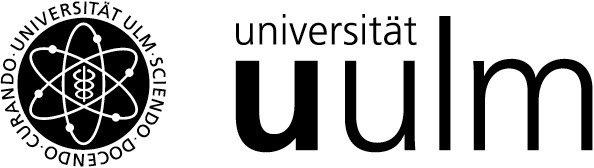
Presentation at the 31st IEEE International Symposium on Computer-Based Medical Systems (CBMS 2018);
Rajeev Motwani, Karlstad, Sweden, 20 June 2018, 15:40 PM