The results of this paper indicate that using movement behavior to predict stress parameters of the TrackYourStress (TYS) users based on machine learning is promising. Yet, this study has several limitations, as explained below. First, the TYS data set is rather small, and the training sample is even smaller. While the choice of as mall sample for training decreases the likelihood of overfitting, it also decreases accuracy. Other forms of sampling could be considered in the future. Second, the TYS mobile apps are not officially released to the App Stores. Following this, the invited TYS users might pose characteristics that bias the generalizability of the presented results. This includes the distribution of the sample data across the quartiles. Third, stress-levels have been designed manually and the features are also handcrafted. So, the relation between features and stress-level is not semantically grounded. Nonetheless, we belied that the results do not justify further investigations on the associations between geospatial and stress data that were gathered by a mHealth crowdsensing platform. In particular, it should be investigated next whether the differences in the obtained accuracies among the quartiles are due to differences in the population distribution, or that they can be explained differently. A further step would be also to monitor stress-levels through sensors (e.g., through the performance of mobile cortisol assessments) rather than resorting to hand-crafted stress-levels. Finally, differences between iOS and Android users in the light of associations between geospatial and stress data seem also to be worth of being investigated.
Machine Learning Findings on Geospatial Data of Users from the TrackYourStress mHealth Crowdsensing Platform
Ulm University Ulm University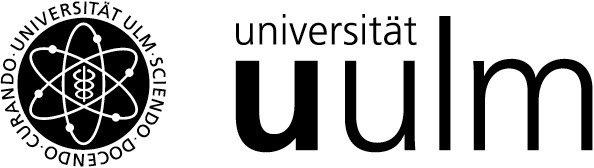
Presentation at the IEEE 20th International Conference on Information Reuse and Integration for Data Science;
Marc Schickler, Los Angeles, USA, 1 August 2019, 4:00 PM