This paper investigates the vision capabilities of multimodal Generative Pre-trained Transformers (GPTs) to auto-generate structured process models from diagram- and text-based documents. We introduce a dataset of 123 process models and corresponding documentation, emphasizing real-world element distributions. Using evaluation metrics for process model similarity, this enables ground truth-based assessment of process model generation. We evaluate commercial GPT capabilities with zero-, one-, and few-shot prompting strategies. Our results indicate that generative vision models can be useful tools for semi-automated process modeling based on multimodal documents.
More importantly, the dataset and evaluation metrics as well as the open-source evaluation code provide a structured framework for continued systematic evaluations moving forward.
Leveraging Generative Vision Models for Extracting Process Models from Documents
Ulm University Ulm University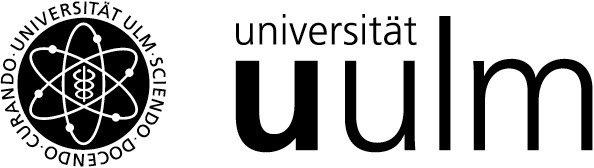
Presentation at BPM 2024; Marius Breitmayer, Krakau, Poland, 02 Sptember 2024