SleepLoop is a Flagship of Hochschulmedizin Zürich and unites researchers from Zurich (ETH Zürich, Universität Zürich, Kinderspital Zürich, Universitätsspital Zürich) and Ulm (Institute of Biomedical Engineering). Our goal is to develop and use technical and clinical innovations to study and improve sleep. We design and research wearables, Big Data, machine learning, and auditory stimulation approaches for this purpose. With this approach we develop and validate better methods for the treatment and prevention of neurodegenerative and systemic diseases.
Why sleep?
Sleep plays a central role in healthy brain and body function. For instance, sleep promotes the consolidation of newly learned information, cleans away toxic waste products in our brain and allows the nerve cells to remain plastic. Consequently, insufficient and poor sleep over longer time periods can have detrimental effects on our health.
How can we improve sleep?
Auditory stimulation during one night of sleep has previously been shown to improve deep sleep in both, young and older adults. The method uses precisely timed tones played during deep sleep, when neurons are most synchronized. Yet, many aspects related to the application of auditory stimulation during sleep remain unclear. To investigate the effects of long-term, remote application of sleep enhancement technologies, we have developed and tested a wearable sleep recording and stimulation device.
How does SleepLoop work?
Our projects
SleepBand
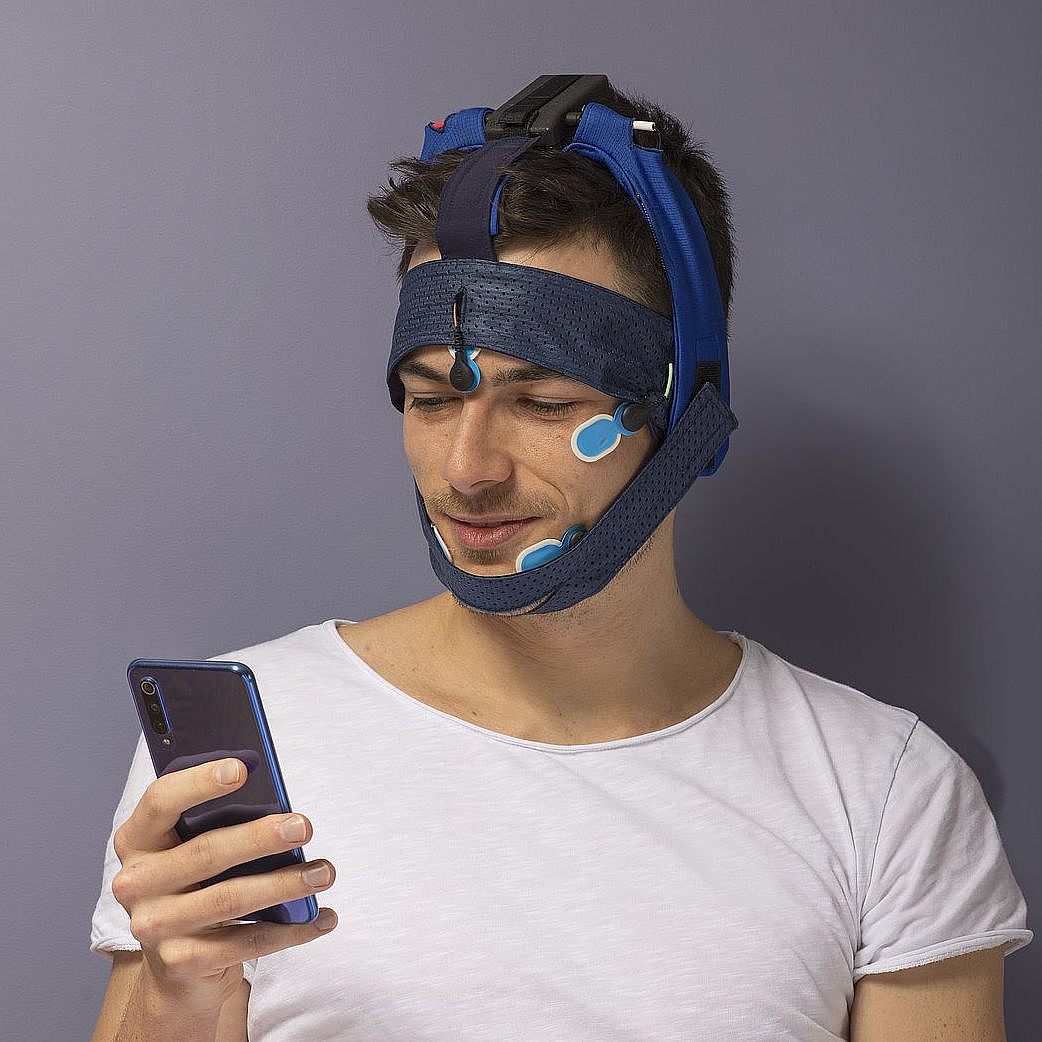
Our mission is to develop wearables with personalized sleep enhancement solutions to enable large-scale research studies and future clinical treatment strategies. With our technology we enable safe brain activity recording and stimulation with superb temporal precision during selected sleep stages. Our autonomous algorithms use the underlying brain activity to control and optimize stimulation to maximally enhance brain activity during deep sleep.
Selected Key features:
- Low-Noise, up to 8-Channel, 24-Bit, Analog-to-Digital Converter for EEG and biopotential measurements
- Ultra-low-power ARM Cortex-M4 for real-time signal processing.
- Rechargeable battery with up to 24 h of recording
- Internet-of-things ready: Bluetooth and Wi-Fi connectivity
- Raw data recording to microSD Card
Publications:
Ferster ML, et al. Configurable Mobile System for Autonomous High-Quality Sleep Monitoring and Closed-Loop Acoustic Stimulation. IEEE Sensors Letters. 2019; 3(5):1--4.
WESA
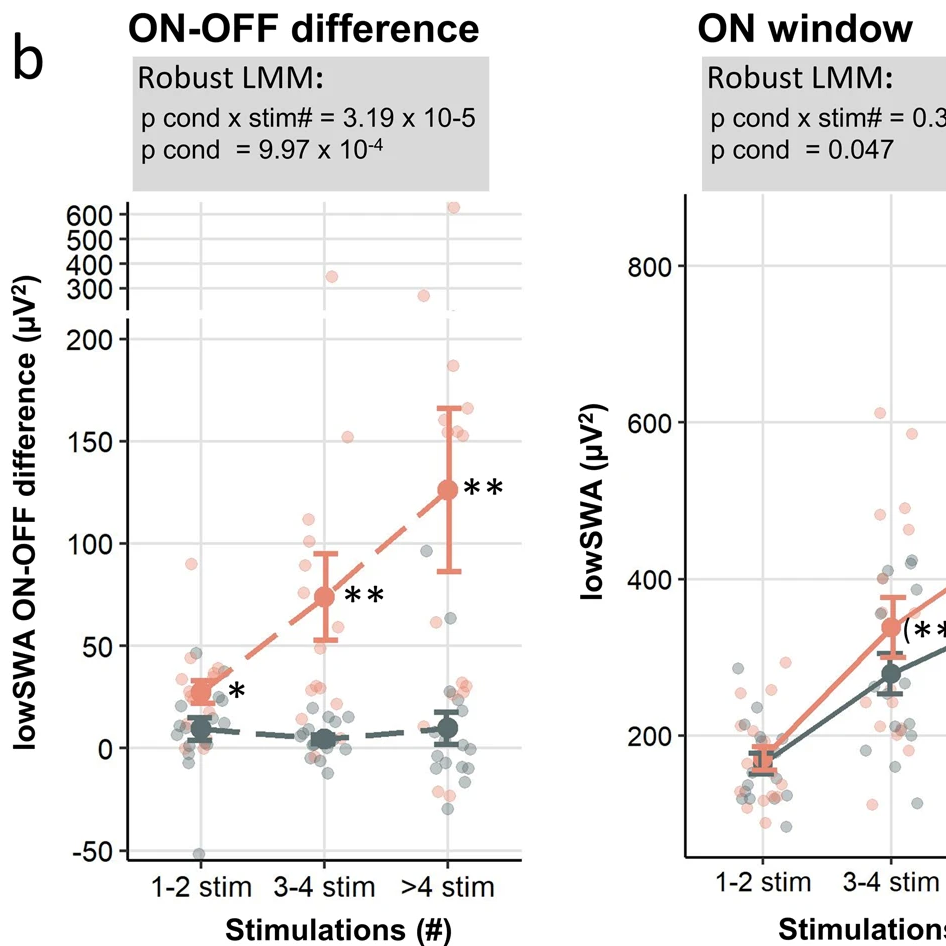
Sleep’s restorative function is closely linked to slow waves, which are brain activity patterns that occur during deep sleep and are diminished with age. Those slow waves can be increased through auditory stimulation, a method that administers precisely-timed sounds during sleep. Here, we established whether the application of auditory stimulation can be performed in older adults over several nights remotely in their own homes. In a trial, we used a mobile device to deliver auditory stimulation during sleep and measured its effects on slow waves, mood, and vigilance. Although we showed robust increases in slow waves, we found large effect differences between participants and also between different nights within the same participants. We looked for predictors of these effect differences. Our study showed that in-home auditory stimulation is feasible, and may help to guide future auditory stimulation strategies.
Publication:
Lustenberger C, et al. Auditory deep sleep stimulation in older adults at home: a randomized crossover trial. Communications Medicine. 2022; 2(1):30. [Doi]
Automated Sleep Classification
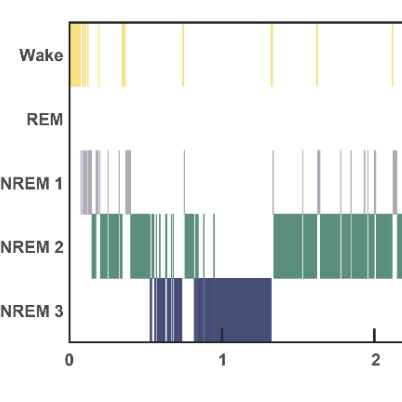
The classification of segments of sleep into one of the five sleep stages – wake, rapid eye movement (REM) sleep , and three non-REM sleep stages – is a standard task in sleep research that is often still performed manually. Distribution of sleep stages enables clinicians and researchers to make valuable observations and assessments regarding sleep architecture, quality and possible disorders. However, the manual sleep classification is labor intensive, costly and can only be applied on selected nights of interest.
To support large studies with automated sleep classification, we have developed a machine learning sleep classification system that is tailored to the SleepLoop recording device. Our method fuses the predictions from multiple deep learning models to produce high-quality labels. Additionally, we investigate alternative classification paradigms such as combining manual and automatic classification in a human-in-the-loop approach.
Our experiments aim to gain a better understanding of the automatic classification quality beyond simple performance metrics, as these are often unsuitable due to the uncertain ground truth present in sleep classification.
Funding
Our projects are /were funded by
- Schweizerische Hirnstiftung,
- Hochschulmedizin Zürich,
- ETH foundation,
- Swiss National Science Foundation
and private Donations.
Partners
Our research collaborators:
- ETH Zurich
- University of Zurich
- University Hospital Zurich
- University Children’s Hospital
- Psychiatric University Clinics of Zurich.