Learning Robots
At the beginning of the project, the range of contents that should be covered in the project will be discussed in detail and the relevant methods will be identified. This is accompanied by a literature search, reading, and discussion phase. In this phase relevant mathematical and computational methods will be selected and discussed. In the second phase, the team starts to implement the experimental design on a simulated robot. Regular team meetings and supervisory consultations lead to an iterated improvement of the software. In the third phase, the thoroughly tested software will be transferred to the robot and tested in a real or simulated environment. The results are written up in a final project report and are presented in a final project presentation.
Dates
- Synchronous online meeting November 19th 2025 6 - 8 pm CET
- Synchronous online meeting December 3rd 2025 6 - 8 pm CET
Synchronous online meeting January 12th 2026 6 - 8 pm CET
The Description of the module you find here.
- Definition of concrete project idea.
- Project plan incl. systematic literature review.
- Evaluation of suitable technologies.
- Self-learning of the required technical foundations.
- Architecture design.
- Implementation.
- Integration.
- Test.
- Deployment.
Students are able to conduct a project-oriented, scientific work in the area of robotics. They have the ability to define an innovative project topic, acquire skills and technologies required for the project, find related works and base their own work on them where appropriate. The students are further able to work independently in a team and to apply state-of-the-art methods to develop concepts and solutions for the project topic. They can document the results in the form of a scientific report and present their findings in the form of a presentation. They are able to acquaint themselves with a new topic and to conduct a scientific project.
The study program combines self-study and group work in a flexible online learning environment. Students have access to video lectures, a detailed and user-friendly script tailored for working professionals, as well as interactive quizzes and exercises. Regular tutorial sessions and online office hours with mentors support the learning process, while discussion forums facilitate exchange among students. For more detailed information, please refer to the module handbook.
Programming in Python or C++, basics of ROS and simulation environments, understanding of machine learning concepts (e.g. Neural Nets, Reinforcement Learning, Computer Vision) may be helpful.
Recommended requirements:
- Desktop computer or notebook, with a supported version of Microsoft Windows, Apple macOS or Linux
- Headset
- Current version of Mozilla Firefox, Google Chrome, Apple Safari or Microsoft Edge
- Access to the internet (e.g., via xDSL, Cable, LTE, 5G) with a minimum data rate of 3 Mbit/s for downstream and 384 kbit/s for upstream.
In case of questions regarding the technical requirements, please don't hesitate to contact us.
Regular participation in online seminars will help you solving exercises, which have to be loaded up to the learning management system after request of the mentor. Passing the exercises successfully is recommended for participation in the final oral examination at Ulm University. For further information, please have a look into the module description.
After finishing your exam successfully you will get a certificate and a supplement, which will list the contents of the module and the competencies you have acquired. The supplement confirms you the equivalent of 3 credit points (ECTS).
Lecturer
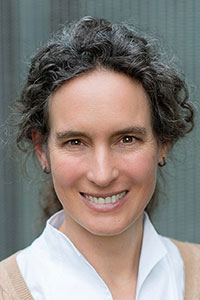
Prof. Dr. Birte Glimm
Chair of the Institute of Artificial Intelligence
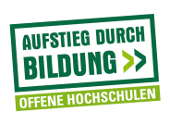
Gefördert von:
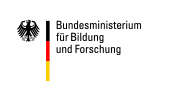
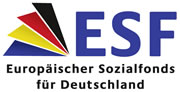
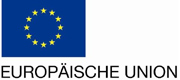